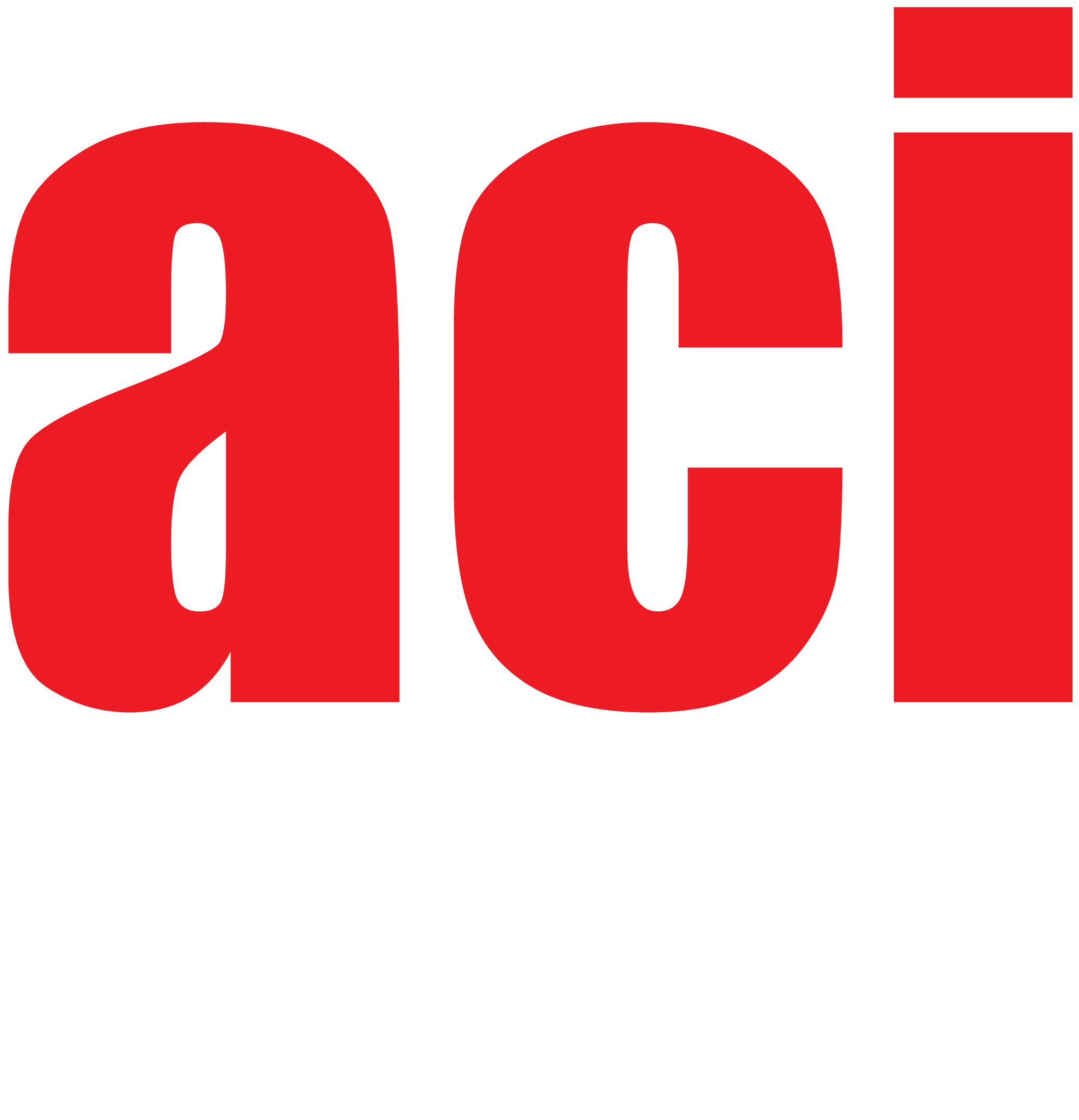
Dado que el uso de simulaciones de elementos finitos (EF) para el análisis dinámico de vigas ferroviarias sobre cimentaciones amortiguadas por fricción (i) requiere mucho tiempo, y (ii) conocimientos técnicos y software avanzados que van más allá de los recursos disponibles de las empresas típicas de ingeniería civil, este artículo pretende demostrar el potencial de las redes neuronales artificiales (RNA) para predecir eficazmente los desplazamientos máximos y la velocidad crítica en vigas ferroviarias sometidas a cargas en movimiento. Se proponen cuatro modelos basados en RNA, uno por rango de velocidad de carga ([50, 175] ∪ [250, 300] m/s; ]175, 250[ m/s) y por tipo de desplazamiento (ascendente o descendente). Cada modelo es función de dos variables independientes, un parámetro de fricción y la velocidad de carga. Entre todos los modelos y los 663 puntos de datos utilizados, se obtuvo un error máximo del 5,4 % al comparar las soluciones basadas en RNA y FE. Mientras que estas últimas implican un tiempo medio de cálculo por punto de datos de miles de segundos, las primeras no necesitan ni un milisegundo. Este estudio supuso un paso importante hacia el desarrollo de modelos basados en RNA más versátiles (es decir, que incluyan otros tipos de variables de entrada) para el mismo tipo de problema.
viewed = 801 timesTambién puede {advancedSearchLink} para este artículo.