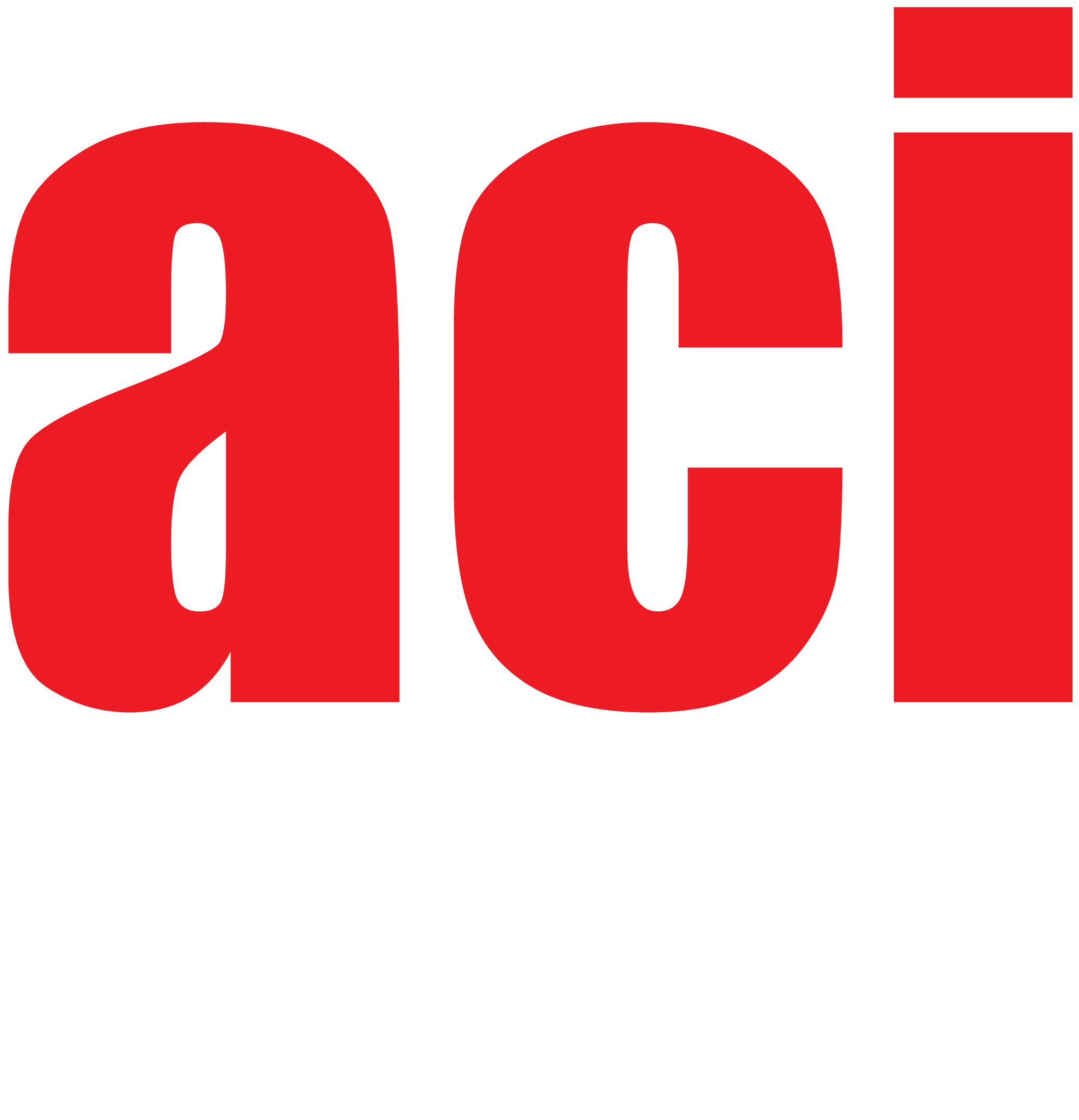
SECCIÓN C: INGENIERÍAS
La tecnología de fabricación y el estado del arte de la ingeniería estructural han conducido a un uso cada vez mayor de estructuras esbeltas, lo que las hace más susceptibles a acciones estáticas y dinámicas que pueden provocar algún tipo de daño. En este contexto, es necesario realizar inspecciones y evaluaciones periódicas para detectar y predecir daños estructurales y establecer acciones de mantenimiento capaces de garantizar la seguridad y durabilidad estructurales con un coste mínimo. Sin embargo, estos procedimientos son tradicionalmente bastante largos y costosos, por lo que son necesarias técnicas que permitan una detección de daños más eficaz. Este trabajo evalúa el potencial de los modelos de Redes Neuronales Artificiales (RNA) en la predicción de la localización de daños en elementos estructurales, en función de sus propiedades dinámicas - se utilizan las tres primeras frecuencias naturales. Basándose en 64 ejemplos numéricos de vigas de acero dañadas (en su mayoría) y no dañadas, se propone un modelo analítico basado en RNA como un estimador de localización de daños altamente preciso y eficiente. El modelo propuesto arrojó errores máximos del 0,2% y el 0,7% en 64 puntos de datos numéricos y 3 puntos de datos experimentales, respectivamente. Debido a la alta calidad de los resultados, el siguiente paso de los autores es la aplicación de enfoques similares a estructuras completas, basadas en conjuntos de datos mucho más grandes.
viewed = 1151 times