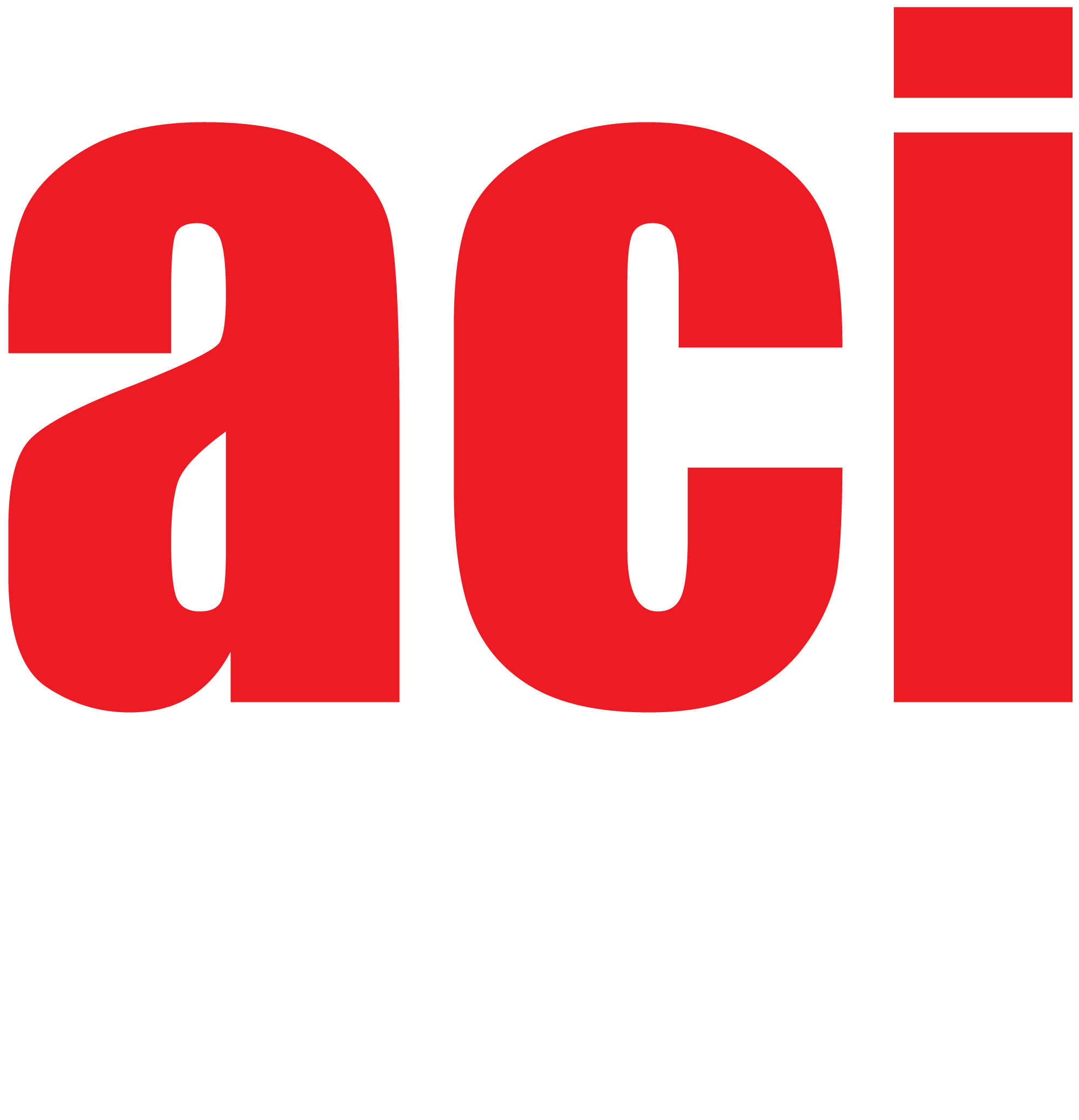
Estimating the balance or vigor in vines, as the yield to pruning weight relation, is a useful parameter that growers use to better prepare for the harvest season and to establish precision agriculture management of the vineyard, achieving specific site planification like pruning, debriefing or budding. Traditionally growers obtain this parameter by first manually weighting the pruned canes during the vineyard dormant season (no leaves); second during the harvest collect the weight of the fruit for the vines evaluated in the first step and then correlate the two measures. Since this is a very manual and time-consuming task, growers usually obtain this number by just taking a couple of samples and extrapolating this value to the entire vineyard, losing all the variability present in theirs fields, which imply loss in information that can lead to specific site management and consequently grape quality and quantity improvement. In this paper we develop a computer vision-based algorithm that is robust to differences in trellis system, varieties and light conditions; to automatically estimate the pruning weight and consequently the variability of vigor inside the lot. The results will be used to improve the way local growers plan the annual winter pruning, advancing in the transformation to precision agriculture. Our proposed solution doesn\textsc{\char13}t require to weight the shoots (also called canes), creating prescription maps (detail instructions for pruning, harvest and other management decisions specific for the location) based in the estimated vigor automatically. Our solution uses Deep Learning (DL) techniques to get the segmentation of the vine trees directly from the image captured on the field during dormant season
viewed = 379 times