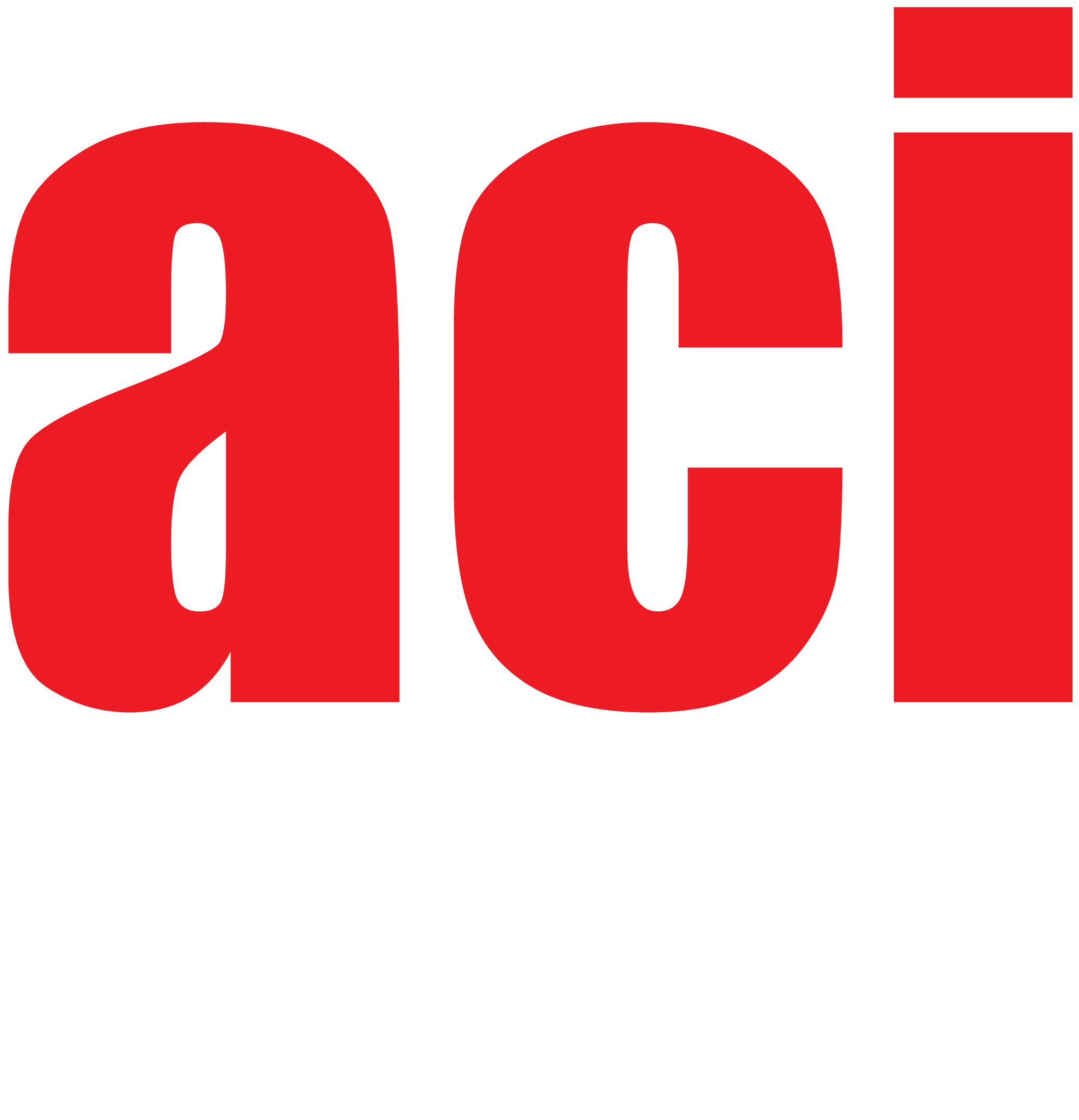
SECCIÓN C: INGENIERÍAS
En el mundo y en el Ecuador, las altas tasas de accidentes de tráfico son generadas, principalmente, por la falta de respeto a la normativa vial por parte de los usuarios viales, generando costos humanos y materiales de importancia. En este sentido, la localización y el reconocimiento de las señales de tráfico es esencial para la construcción de dispositivos capaces de prevenir situaciones que puedan generar accidentes de tráfico. Por lo tanto, en este trabajo se presenta un nuevo enfoque para la construcción de un sistema para la detección de señales de tráfico, usando las siguientes innovaciones, i) un método de segmentación por color para la generación de regiones de interés (ROIs) basado en los algoritmos ð‘˜âˆ’ð‘ð‘, con ð¾ð‘šâˆ’ð‘šð‘’ð‘Žð‘›ð‘ , ii) una nueva versión del descriptor HOG (Histograms of oriented gradients) para la extracción de características, y iii) el entrenamiento del algoritmo SVM no-lineal para multi-clasificación. El enfoque propuesto ha sido probado sobre las señales de tráfico ecuatorianas de Pare y Ceda el Paso. Un sinnúmero de experimentos han sido desarrollados sobre varias secuencias de videos capturadas con vehículo experimental en condiciones reales de conducción en varias ciudades ecuatorianas, bajo diferentes condiciones de iluminación como son normal, soleado y nublado. Este sistema ha mostrado un rendimiento de 98.7% para la segmentación, 99.49% para la clasificación y una precisión del 96% para la detección.
viewed = 712 times