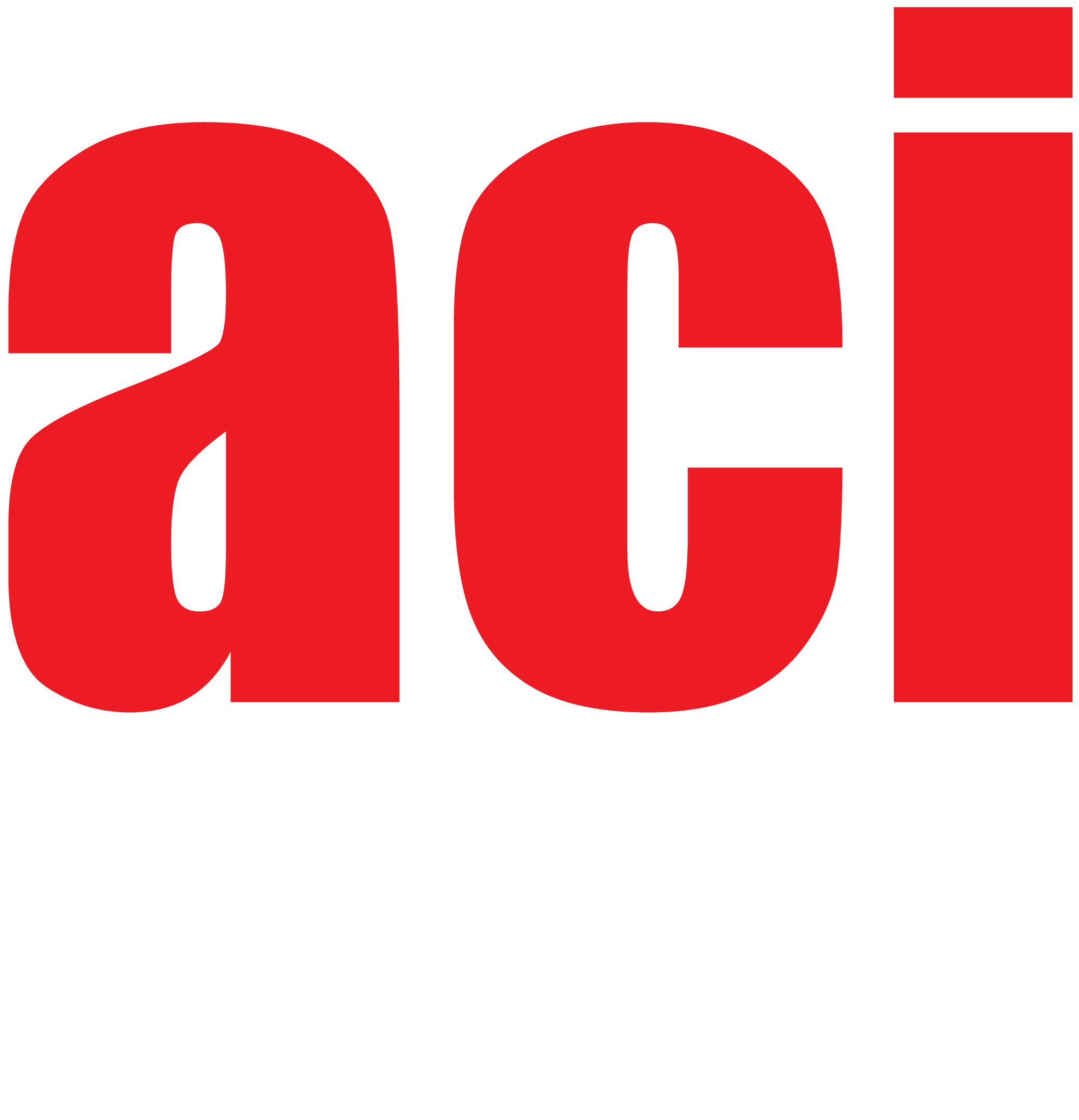
Los bifenilos policlorados (PCBs) son contaminantes persistentes que afectan enormemente a los ecosistemas marinos. Utilizando técnicas de aprendizaje de máquina, se construyeron modelos de relación cuantitativa estructura-actividad (RCEA) para predecir el factor de bioconcentración (BCF) de los PCBs. Estos modelos se construyeron a partir de descriptores topográficos 2D y 3D calculados para la estructura molecular optimizada en el nivel de mecánica molecular. Después de analizar sus parámetros estadísticos, se determinó que dos modelos son bastante robustos para la predicción de logBCF. Los modelos seleccionados fueron: M_4_LR construido con dos descriptores moleculares y presenta valores de r2 = 0,9154, Q2LOO = 0,8944, y Q2ext = 0,9119, y M_13 construido con cuatro descriptores moleculares y presenta valores de r2 = 0,9375, Q2LOO = 0,9155, y Q2ext = 0,844. Los dos modelos pasaron la doble fase de validación y cumplieron con los criterios de la prueba de Tropsha. Esto implica que las predicciones para el logBCF fueron bastante precisas tal como se muestra en los resultados del presente estudio.
viewed = 631 times