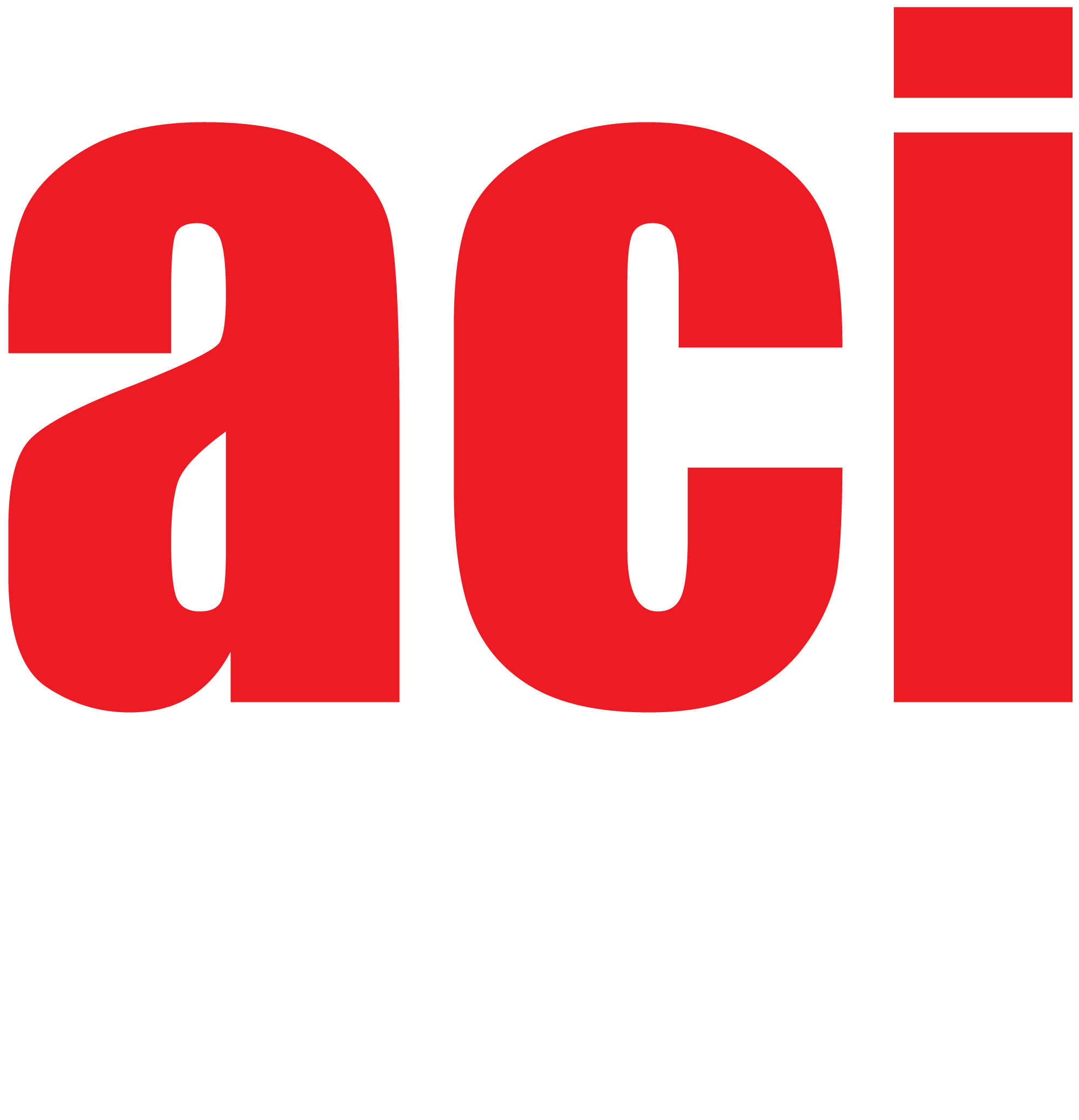
SECTION B: LIFE SCIENCES
The absence of time series data on meteorological variables is a drawback in environmental sciences, especially with regard to precipitation, which is a key variable in several fields. The present study aimed to compare several statistical and mathematical methods to generate missing pluviometric data in the microbasin of the Pita River, such as the Paulhus and Kohler method, multiple linear regression (MLR), Wavelet transform and artificial neural networks, using information from the hydrometeorological network of the Fund for Water Protection (FONAG) of Quito.
viewed = 268 times