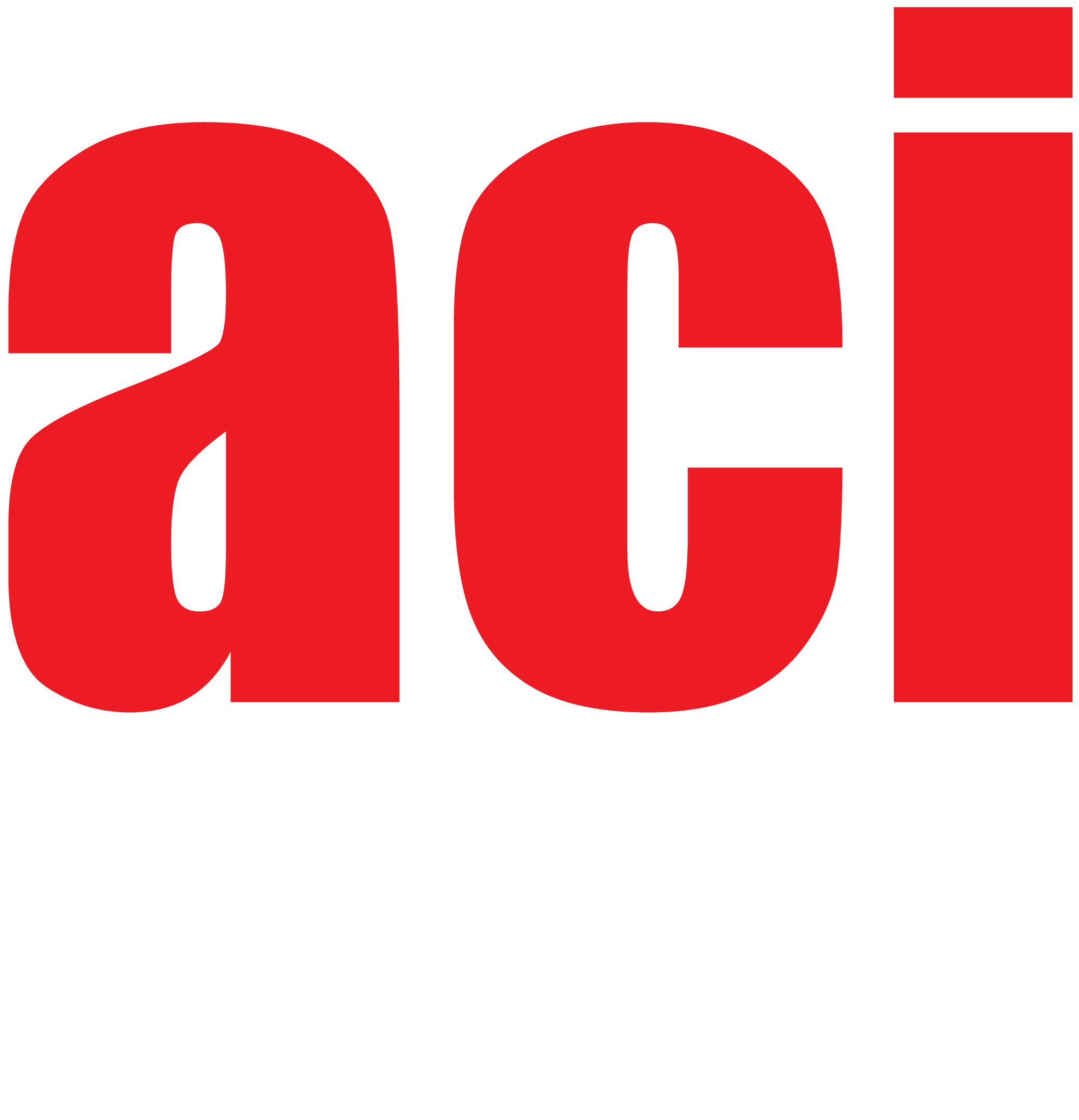
Numerosos trabajos han mostrado que las distribuciones de probabilidad caracterizadas por la media y varianza resultan inadecuadas para expresar regularidades asociadas a variables de tipo geográfico o espacial. Esto se debe a que los datos no se aglomeran alrededor de un valor central; más bien, las colas se vuelven pesadas y los eventos extremos resultan ser menos improbables que a partir de otras distribuciones. Bajo esta consideración, el presente trabajo apunta a encontrar leyes empíricas sobre algunas variables relevantes de ciudades de Ecuador, Para ello, se parte de dos hipótesis: 1) ciertas variables socioeconómicas se escalan a través de una variable de tamaño y 2) dichas variables se distribuyen bajo una ley potencia; se estiman los parámetros requeridos y se contrastan otras distribuciones de cola pesada que ajusten adecuadamente los datos escogidos.
viewed = 637 times