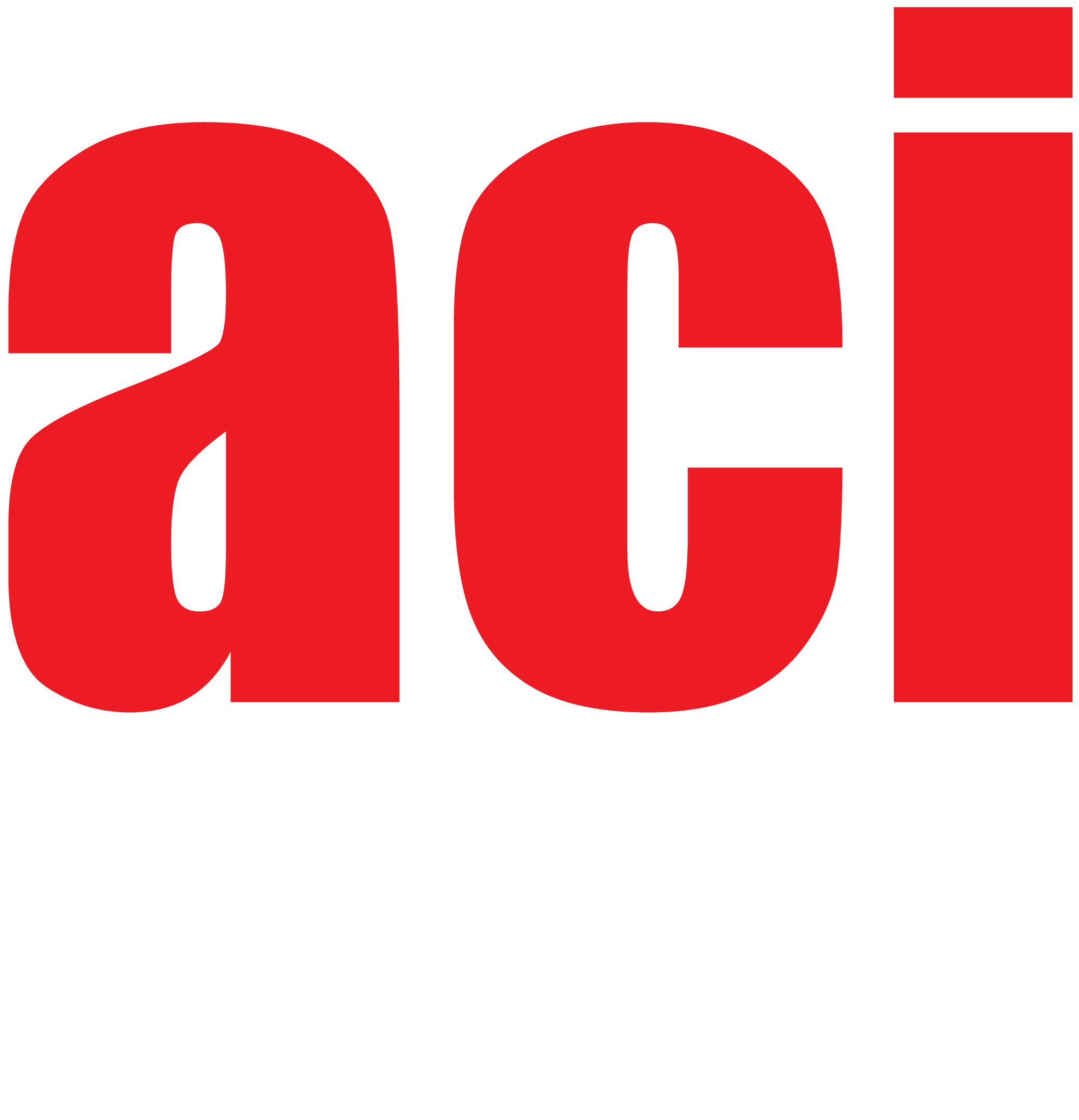
Los testores, y en particular los testores típicos, han sido utilizados en problemas de selección de variable y problemas de clasificación supervisada. Comunmente se ha usado algoritmos determinísticos para hallar testores típicos. A principios de esta decada comenzó a desarrollarse un nuevo enfoque basado en algoritmos evolutivos. Un problema común para probar el comportamiento de ambos métodos es la necesidad de conocer a priori el número de testores típicos de una matriz dada. Para una matriz arbitraria, no se puede saber este número a menos de que se hayan encontrado todos los testores típicos. Por lo tanto, este trabajo introduce, por primera vez, una estrategia para generar matrices básicas para las cuales el número de testores típicos es conocido sin necesidad de aplicar un algoritmo para encontrarlos. Este método se ilustra con algunos ejemplos.
viewed = 819 times